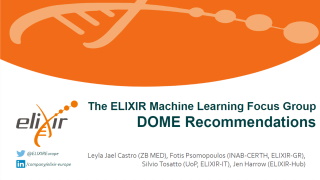 | ELIXIR ML Focus Group More information on the web page Currently co-chaired by Fotis Psomopoulos, Silvio Tossato, Leyla Jael Castro |
| Why do we need standards to publish machine learning approaches? Machine/Deep learning has a high impact in biomedical research, it touches all areas. We have more and more publications but there is no agreement on what key elements could be use to describe a machine learning approach For instance, about 20% of publications including a machine learning approach do not include information about the evaluation |
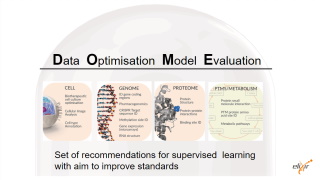 | The DOME recommendations propose some of those key elements for machine learning approaches They include Data, Optimization, Model and Evaluation By now, they focus on supervised learning only |
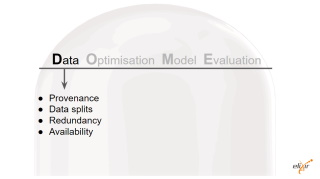 | - Provenance: Protein Data Bank (PDB). X-ray structures missing residues. Npos = 339,603 residues. Nneg = 6,168,717 residues. Previously used in (Walsh et al., Bioinformatics 2015) as an independent benchmark set.
- Dataset splits: training set: N/A. Npos,test = 339,603 residues. Nneg,test = 6,168,717 residues. No validation set. 5.22% positives on the test set.
- Redundancy between data splits: Not applicable. Availability of data Yes, URL: http://protein.bio.unipd.it/mobidblite/. Free use license.
|
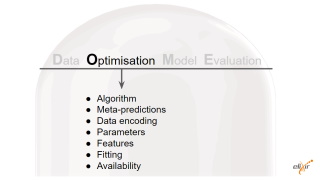 | - Algorithm: Majority-based consensus classification based on 8 primary ML methods and post-processing.
- Meta-predictions: Yes, predictor output is a binary prediction computed from the consensus of other methods; Independence of training sets of other methods with test set of meta-predictor was not tested since datasets from other methods were not available.
- Data encoding: Label-wise average of 8 binary predictions.
- Parameters: p = 3 (Consensus score threshold, expansion-erosion window, length threshold). No optimization.
- Features: Not applicable.
- Fitting: Single input ML methods are used with default parameters. Optimization is a simple majority.
- Regularization: No.
- Availability of configuration: Not applicable.
|
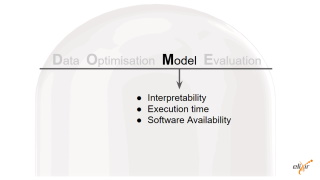 | - Interpretability: Transparent, in so far as meta-prediction is concerned. Consensus and post processing over other methods predictions (which are mostly balck boxes). No attempt was made to make the meta-prediction a black box.
- Output: Classification, i.e. residues thought to be disordered.
- Execution time: ca. 1 second per representative on a desktop PC.
- Availability of software: Yes, URL: http://protein.bio.unipd.it/mobidblite/. Bespoke license free for academic use.
|
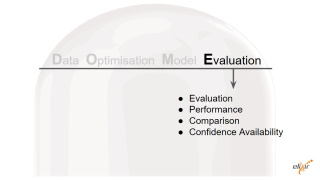 | - Evaluation method: Independent dataset
- Performance measures: Balanced Accuracy, Precision, Sensitivity, Specificity, F1, MCC.
- Comparison: DisEmbl-465, DisEmbl-HL, ESpritz Disprot, ESpritz NMR, ESpritz Xray, Globplot, IUPred long, IUPred short, VSL2b. Chosen methods are the methods from which the meta prediction is obtained.
- Confidence: Not calculated.
- Availability of evaluation: No.
|
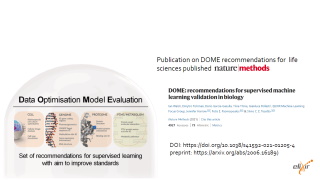 | You can find more information in the published paper and the DOME website |
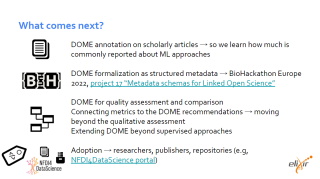 | - DOME annotation on scholarly articles → so we learn how much is commonly reported about ML approaches
- DOME formalization as structured metadata → BioHackathon Europe 2022, project 17 “Metadata schemas for Linked Open Science”
- (Metadata) DOME for quality assessment and comparison
- (Metadata) Connecting metrics to the DOME recommendations → moving beyond the qualitative assessment
- (Metadata) Extending DOME beyond supervised approaches
- Adoption → researchers, publishers, repositories (e.g, NFDI4DataScience portal)
|